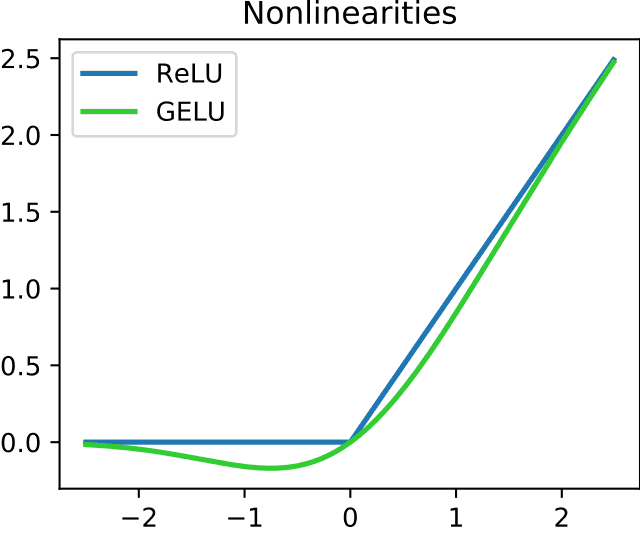
Rectifier (neural networks)
Activation function / From Wikipedia, the free encyclopedia
Dear Wikiwand AI, let's keep it short by simply answering these key questions:
Can you list the top facts and stats about Rectifier (neural networks)?
Summarize this article for a 10 year old
In the context of artificial neural networks, the rectifier or ReLU (rectified linear unit) activation function[1][2] is an activation function defined as the positive part of its argument:

where x is the input to a neuron. This is also known as a ramp function and is analogous to half-wave rectification in electrical engineering. This activation function was introduced by Kunihiko Fukushima in 1969 in the context of visual feature extraction in hierarchical neural networks.[3][4][5] It was later argued that it has strong biological motivations and mathematical justifications.[6][7] In 2011 it was found to enable better training of deeper networks,[8] compared to the widely used activation functions prior to 2011, e.g., the logistic sigmoid (which is inspired by probability theory; see logistic regression) and its more practical[9] counterpart, the hyperbolic tangent. The rectifier is, as of 2017[update], the most popular activation function for deep neural networks.[10]
Rectified linear units find applications in computer vision[8] and speech recognition[11][12] using deep neural nets and computational neuroscience.[13][14][15]